10 Common Data Management Mistakes
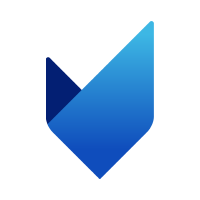
As big data applications are expanding at a much faster pace, more and more businesses are choosing the path of digital transformation to maintain relevancy and stay abreast with the current trends. It has been seen that organizations have recognized the importance of big data and are treating data as an asset (probably one of the most valuable of all due to its ability to decide growth trajectory and ability to offer a competitive advantage over competitors), but have failed to draw any fruitful insights from it.
It’s true that the ability to leverage data isn’t everybody’s forte and transforming it into information that is consistent, correct, and comprehensive is often found to be missing. Wondering what businesses should do in such a scenario? Technology honchos favor data governance program that allows data to be treated as a corporate asset by imposing rules, regulations, policies, and procedures but it’s often easier said than done.
In recent times, many businesses around the globe have ramped up their enterprise data governance efforts but looking at the success rate of these initiatives has been disheartening. Those of you who are involved with big data would agree that businesses face tough times when it comes to the massive amount of data that need to be controlled and made sense to maintain competitiveness, meet customer needs and most importantly, comply with the law. The struggle to architect programs that enable sustainability offer organizational credence and decide the business integrity is real.
Growing Data Requires Better Data Management
According to Infosys, the world of structured data is growing by over 40 percent every year, unstructured data at 80 percent, and global data is expected to touch 40 zettabytes by next year. Recently Forbes said that organizations are keen to spend on big data app development to manage the huge volume of information created where 40 percent of these apps are customer-facing. While these figures prove the fact right that big data is huge and it can do wonders for a business, its volatility can’t be ignored, and the road from adoption to action is actually rocky.
Investing in big data is definitely lucrative, but unplanned management can result in a huge mess. Avoiding such a situation is possible when you are aware of the common mistakes in advance. Below is a handy checklist of a few of the common data management mistakes that businesses should avoid to maximize the full potential of big data implementation.
1. The Absence of a Data Governance Body to Manage the Administration
Before proceeding with the implementation, the onus lies with the organization to ensure that an effective data governance framework is put in place to keep the complete life-cycle in check. This becomes possible only when a governing authority is formed comprising the right kind of skilled individuals who can oversee proper data administration. In case of any mishap, this governing body will have the authority to seek answers from those employees who are part of the data governance project. Under no circumstances should this step be missed as it ensures complete control over the implementation process.
2. Not Paying Attention to Data Architecture
Wondering what it means? Well, this tends to manifest itself in the below-mentioned ways
- Making no investments in architecture and its tools
- Limited investments with periodic reviews but lack dedicated practice
- Absence of integration between architecture and other essential processes like portfolio management
- Having no architectural methodology in place
You will be able to bag maximum benefit out of it when you choose to invest in it. When you use fewer resources and not completely committed toward it, the value derived will obviously be less.
3. Treating Data Governance as a Project
It has been observed that many organizations tend to treat data governance initiative as a traditional project. Since data is dynamic, ever-changing, and has many touch points, the traditional project management approach isn’t the right fit for data governance. The program approach is the best fit for data governance in which one would be allowed to define a series of project streams that focus on one key area.
As long as new data is flowing in and out of the organization, data governing should be a continuous process.
4. Ignoring the Data Quality
Like it or not, data consistency and accuracy drives the success of a data governance initiative. Data integrity can be ensured when sufficient measures are in place. Business decisions are data-driven, and if the quality of data is questionable, it hampers the company’s decisions. It is a must to have correct and trustworthy data to draw insights from to ensure informed business decisions are made. When data quality is maintained within every application, standardized data evaluation becomes reality.
5. Following the Silo Approach in Data Governance
Although a data governance program limited to a particular business unit may help that unit, problems crop up since data sharing happens across varied business groups where each group sets the definition of a specific data element accordingly. This can lead to poor decisions. Ensuring data governance success is only possible when a business treats data as an organizational asset. A “think globally, act locally” approach is what organizations need to follow.
6. Practicing Poor Data Profiling
Data profiling is an absolute must for developing world-class data integration applications. It is a common practice followed by the ETL developers to look at the present dataset and develop the ETL to clean and process the dataset. Unfortunately, that is not enough.
To understand this better with an example. Say, for instance, customer “X” is found with the postal code in the city field, and then we add an instruction in the ETL for that particular “X” customer to extract the postal code from the city field and place it in the postal code field. For the current dataset, this works, but what if in the future, another customer (e.g. customer “Y”) does the same thing? This example shows that not taking your future datasets into account means it only supports your present dataset. As data is unpredictable and can change anytime, flexibility in the dataset is essential. In-depth data profiling right at the start of the project ensures that less time is spent to make updates to the data cleaning portion of the ETL in the future.
7. Collected Unnecessary Data
One of the key features of data governance is a good data retirement strategy. Not sure what it is all about? At some point in time, every data should be recycled. But when organizations do not follow this approach, it means that they need extra cycles just to ensure every data is in order. According to the Veritas Global Databerg Report, 85 percent of stored data is either dark or redundant, obsolete, or trivial (ROT).
8. Piling up Unmaintained Applications
If an app has some flaws or grey areas and you continue working on it, their complexity will escalate further in the near future. Soon or later, the software will fail to deliver and you may be left with the only option to redevelop it right from scratch. This situation can be avoided when you ensure that the applications are maintained and updated on time without fail.
9. Looking Just to Meet Compliance Target
When an organization is asked to follow data governance just to meet the regulator’s requirements, in such a scenario a business is most likely to opt for shortcuts. When the focus is placed on satisfying the regulator’s demands instead of achieving the long-term goals, business benefits take back seat.
10. Relying Solely on the IT Team
Many a time, organizations ask the IT team to handle and manage the data governance initiatives. While it may seem logical to assign IT to lead the data governance functions, it is equally imperative to consider other business processes to ensure a well-balanced approach is maintained.
Start Small, Take Baby Steps
When embarking on data management, the key to success lies in the belief that it is an ongoing process and hence start small. So, instead of focusing on building databases and data management systems, make them work together to boost efficiencies.
Aiming to resolve all your data problems in the initial phase of data management invites trouble. We would suggest that you go slow and take baby steps to avoid pitfalls and meet your organizational demands on time. If you are not sure how to proceed with the whole process, it is best to collaborate with a solution provider who is able to direct you in the right direction.
PKWARE can help. Start here with a free demo.