The Difficult Reality of Data Discovery – The Structured Data View
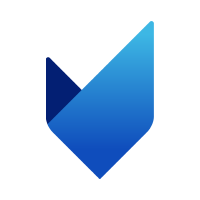
Data Discovery has been around for many years, with many use cases, such as helping organizations analyze data for actionable insights and to help lower corporate risk. It has traditionally been a function of information security. The term refers to the identification of data elements in a repository in order to inventory and protect the data based on its sensitivity.
It has only been in the past couple of years that privacy professionals discovered (no pun intended) the importance of effective data discovery for adequately meeting regulatory requirements. This ongoing shift in viewing discovery as a foundational requirement for privacy compliance has had a profound impact on the discovery field. When data discovery was used for data protection purposes, companies focused on a limited set of sensitive elements, such as government-issued identifiers and financial information, as those were commonly targeted for abuse. When used for privacy compliance, discovery requires a much broader list of data elements to be identified, and requires the use of those elements to correctly match the identities of data subjects as well.
Finding the Right Data
Data discovery is not about perfection—it is about decreasing the instances of false-positive and false-negative identification. In short, the false-positive identification of data means that a data element is incorrectly identified as meaning X when in fact, it means Y. False-negative identification means that the data element of interest that exists in the data set is not discovered. Unfortunately, technology today never completely eliminates false-positive and false-negative identification; however, the ability to be as accurate as possible has become one of the key differentiators between discovery solutions in the market.
Common Discovery Challenges with Structured Data
There are many reasons why data discovery is a challenge that requires the right technology, coupled with experience and expertise. Below, you will find a short list of examples outlining common challenges when conducting data discovery in databases with structured data.
- Table Headers – Ideally, the headers of database tables will have descriptive titles that clearly identify the content, supported by additional documentation that further describes the data and the relationship between tables. This ideal state is not as common as organizations would like. For many years, database administrators were the only ones who needed to access the tables, and therefore, creating clear, descriptive headers was simply not a priority.
- Keys as Attributes – Another unique challenge tied to the problem of poorly titled table headers is the use of values as a stand-in for the real attribute. For example, when indicating whether a certain data subject has opted in or out of a certain activity, the opt-in response may be represented as the number one and opt-out as number two. Today, there is no way any tool, human or artificial intelligence, can make sense of this column of ones and twos without proper documentation.
- Data Quality – The data we encounter in repositories varies greatly in its completeness, accuracy, and relevance. These aspects of data quality impede a tool’s ability to make sense of data and adds time to the discovery process. It is important to note that for efficiency, data discovery tools often sample the data rather than read it in its entirety. When the data quality is poor, the tools must be adjusted to use larger samples and consequently further delays the discovery process.
- Regional Implications on the Same Data Element – Each country has a different way of writing physical addresses, national identification numbers, passport numbers, phone numbers, etc. In the US, there are over 50 different types of driver license numbers; in Canada, there are nine (one for each of its provinces). A column in a table that contains a variety of passport numbers is not likely to be identified as such if the discovery tool is not “trained” to recognize these variations.
- Diversity of Systems – Discovery of data across the enterprise means scanning different types of repository technologies. The diversity of systems is a real market challenge because companies regularly grow through acquisition and mergers, in which they consequently bring more technologies under their roof. An effective discovery technology must be able to scan a system-diverse enterprise with a consistent level of results (easier said than done).
Of course, there are many other obstacles to effective sensitive data discovery as well. Knowing what data and data subjects exist across the enterprise is the foundation on which privacy operations and data protection rest. Without it, complying with regulatory requirements is more of a guess than a well-controlled process.
Learn more about how PKWARE supports accurate discovery with PK Discovery, then see it in action with a free demo.