Would You Put Your Data in the Hands of an AI?
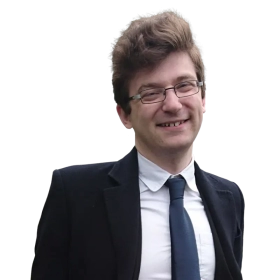
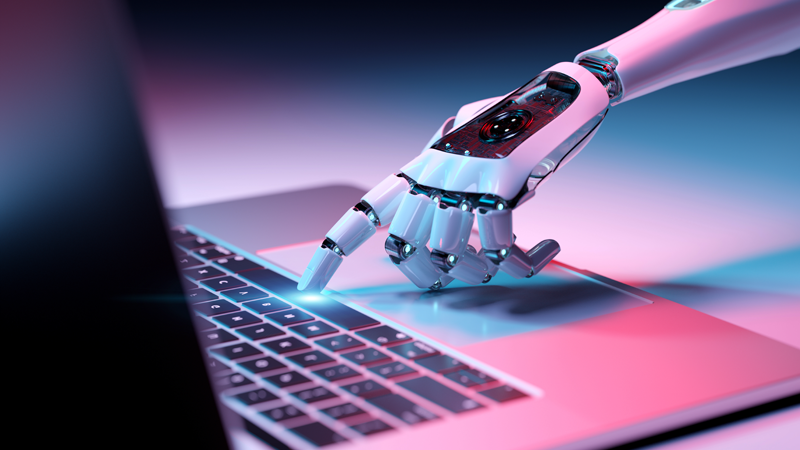
featuring guest author Daniel Howard, Senior Analyst, Bloor Research
AI is one of the more recent hot button issues to spring out of the tech world and capture the imagination (and sometimes consternation) of the general public. Actually, that’s only half true: AI’s been the subject of sci-fi media and particularly nerdy dinner party conversations for decades. But there has been a significant upswell in both excitement and concern about AI in recent years, by both businesses and the general public. This is largely due to significant advances in the use of machine learning (ML) technologies, and most recently by the advent of publicly available generative AI engines such as ChatGPT. The funny thing is that the tech that underpins this sort of technology isn’t really that new, but now that the general public has got its hands on it, mass media has taken an interest, and here we are.
To underscore just how popular this sort of thing has become, a few weeks ago I was out having a drink and talking about AI with a colleague, only for our conversation to be overheard and interrupted by a total stranger who felt the need to chip in their own opinion on the topic. I think we can all agree that when random people (and especially random British people—as a nationality we are infamously poor at talking to strangers) start accosting you with their opinions on AI, it has well and truly become a conversation that everybody wants to be a part of.
Back in the world of business, AI in general and generative AI in particular offer significant benefits as well as significant challenges for data, especially in terms of data governance and security. But how exactly does this manifest?
The Benefits of AI in Business and Data Governance
First, the good: AI (or rather, ML) is increasingly in use as an advanced form of predictive analytics. This is well-known – data science is hardly a new field. But both the sophistication of the technology and the appetite for it have been growing for years. For data governance in particular, this can be applied to the process of discovering and classifying your data, and sensitive/personal data in particular.
One of the perennial problems for sensitive data discovery is the presence of false positives (and to a lesser extent, false negatives). False negatives are usually completely unacceptable (it means you have undetected—and therefore vulnerable—sensitive data floating around somewhere in your system) which means you need thorough systems for detecting sensitive data. But at the same time, data that is flagged as sensitive unnecessarily will need a human to reclassify it so that it doesn’t get put through a masking or encryption pipeline for no reason. If you get a lot of false positives from your discovery tests, this can end up as a lot of manual work. So the question is: How do you make sure you’re capturing all of your sensitive data while minimising the amount of non-sensitive data that is mistakenly tagged as sensitive by your discovery algorithms?
AI is (the start of) a good answer. Although a variety of matching techniques are available to discover sensitive data (column name matching, dictionary matching, pattern matching, proximity matching, RegEx, Natural Language Processing (NLP)—the list goes on) almost all of the more sophisticated techniques can either be enhanced by or rely on AI-driven technologies (usually ML) to intelligently detect and pare down false positives.
This kind of AI use can also apply to the more general classification of data, whether sensitive or non-sensitive, which is often used as part of data governance efforts (to feed a data catalogue, for example). It is also worth mentioning AI’s capability to improve in accuracy over time as it “learns” your system.
Challenges Still Loom
Now, the bad. There are several fairly well-known challenges to implementing AI via ML, including the need to train your AI models in order to maximise their effectiveness (creating a lag time before that can be achieved), the fact that predictive models can only ever be as good as the data you train them on (and hence can absorb biases that are present in that data—just run a search on “racist AI” to see the kind of thing that can lead to), and the voluminous nature of ML model architectures (Champion-Challenger, for instance) meaning you have to figure out a way to manage hundreds (or thousands, or tens of thousands) of models en masse. But all of these problems have been around for a while, and they are generally addressable, to one extent or another, using the technology available today.
Generative AI is a different story. Although the tech, as already discussed, isn’t quite as new as the headlines might suggest, the public awareness and adoption of it certainly is. As such, there are a number of potential problems it might cause simply because no-one has really been thinking about them until recently.
For instance, it’s easy to imagine ChatGPT and its ilk being harnessed to write a huge number of highly convincing phishing emails, either by independent hacking groups or by hostile governments (which ones those might be is left as an exercise to the reader). This is concerning, because social engineering (of which phishing is a major subcategory) is already by far the most successful method to circumvent cybersecurity measures and get access to and expose sensitive data. Being able to crank out the material for those attacks automatically and in massive numbers is sure to make the problem significantly worse. This makes it more important than ever to find and protect your sensitive data, both via technology such as masking and through training privileged individuals in good security practices, like how to detect phishing and other forms of social engineering.
There is also a concern that generative AI engines could expose real, sensitive data if asked the right questions. What’s more, AI engines are designed to learn from their inputs. What if someone (mistakenly or deliberately) just tells the engine some sensitive info? Will the AI be sophisticated enough to realise that data is sensitive and that it shouldn’t reproduce it? While there are safeguards in place on some engines to prevent this sort of thing, it’s not yet clear how effective they will be when really put to the test (in an enterprise environment, say).
Verdict: Balance is Key
To conclude, ML as it currently stands—which includes generative AI—is a sophisticated and useful tool, and it must be assessed as such from a business perspective.* As with any such tool, it will surely cause both great successes and great problems for data governance, data security, and data in general.
*The social issues raised by AI, especially generative AI, are for my money very real (will AI art put artists out of business? Yes, probably) but outside the scope of both this paper and, frankly, my expertise. This goes double for the philosophical questions that surround the field.
Read more from Bloor Research: Sensitive Data Discovery Market Update
- Tait Hamiel May 16, 2025